Experimental Study of Inequality and Unpredictability in an Artificial Cultural Market
postMatthew J. Salganik,Peter Sheridan Dodds, Duncan J. Watts
Published: 2006-02-10
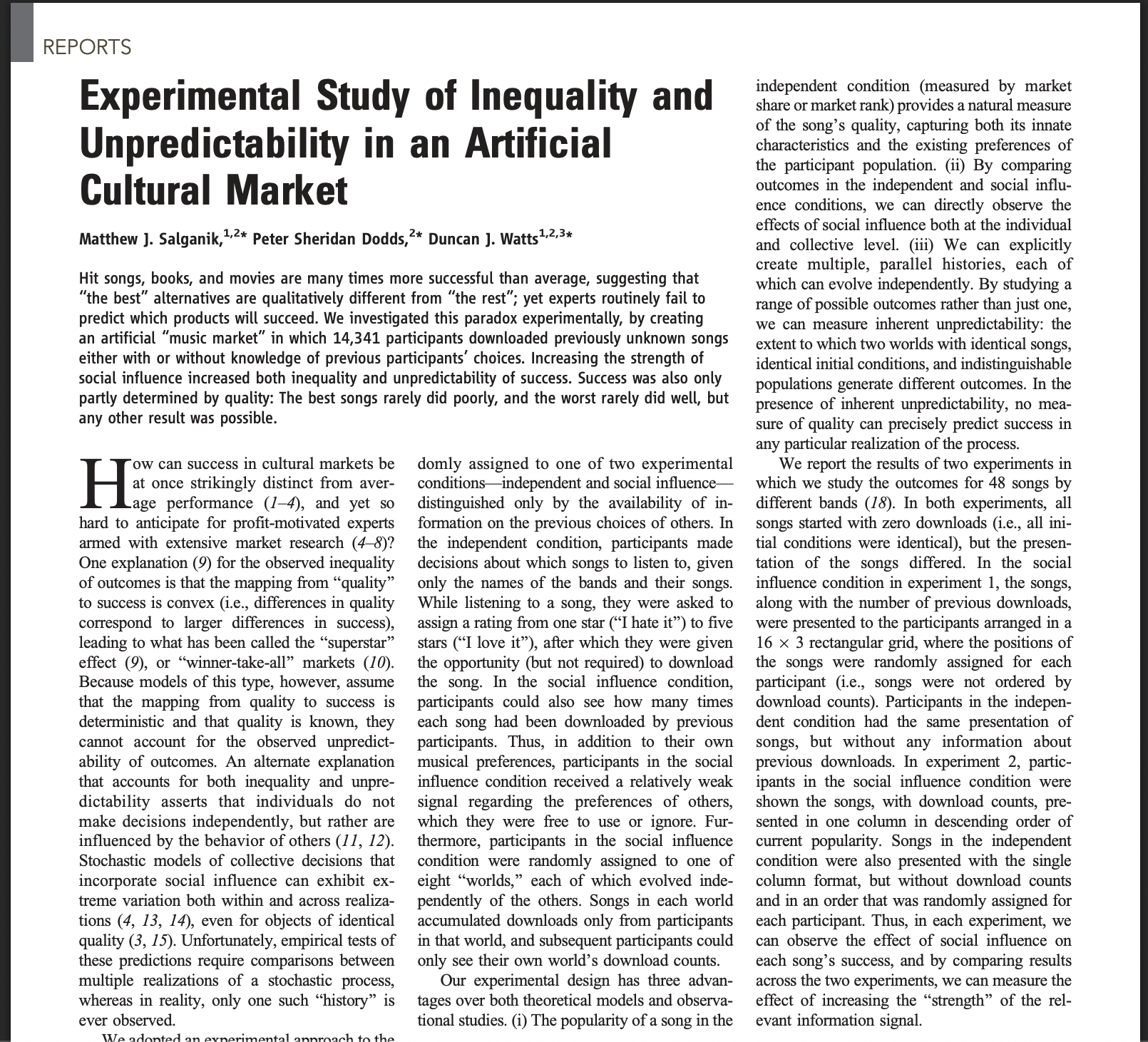
🔥 Key Takeaway:
The same product can become both a massive hit and a total flop—purely due to social influence—so the “best” idea doesn’t always win, and success is often more about the crowd’s unpredictable feedback loop than the quality of what you’re selling.
đź”® TLDR
This 2006 Science paper by Salganik, Dodds, and Watts tested how social influence shapes market outcomes by running a large-scale artificial music market with 14,341 people. When participants could see what others downloaded, popular songs got much more popular while others faded, leading to high inequality, and which songs became hits was unpredictable—even with identical starting conditions and song lists. Only the very best and very worst songs had reliably consistent outcomes; for most, success or failure varied widely across parallel "worlds" purely due to social effects. Making social signals (like download counts) more prominent further increased both inequality and unpredictability. The key takeaway is that social influence alone can drive both runaway popularity and randomness, so any attempt to simulate or predict real-world market success must model these social dynamics directly—assuming outcomes are determined just by quality or individual preference misses the core driver of observed market volatility and hit formation.
📊 Cool Story, Needs a Graph
Figure 1: "Inequality of success for social influence (dark bars) and independent (light bars) worlds for (A) experiment 1 and (B) experiment 2."
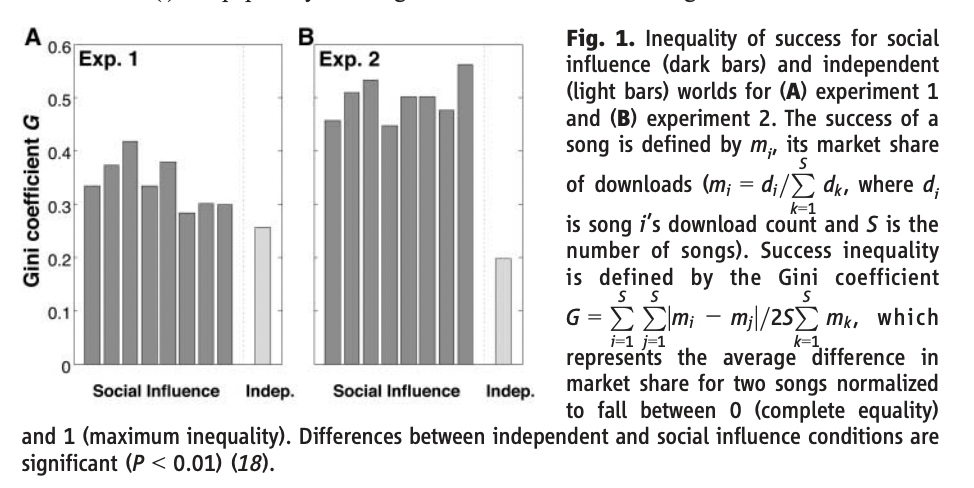
Social influence significantly increases market inequality compared to independent choice, and stronger social signals amplify this effect. Figure 1 shows side-by-side bar charts comparing the Gini coefficient—a standard measure of inequality—for song download outcomes in both the "independent" condition (where participants make choices without seeing others' behavior) and the "social influence" condition (where download counts are visible). Across two experiments, all eight social influence worlds consistently show higher inequality than the independent baseline, with inequality rising further when the social signal is made more prominent (as in experiment 2). This visually demonstrates that adding social influence causes a few songs to become disproportionately popular, while most are ignored, and that this effect scales with the strength of the social information provided.
⚔️ The Operators Edge
A crucial but easy-to-miss detail is that the experiment’s unpredictability and runaway winner effects only showed up because the researchers created *multiple, truly independent parallel worlds*—not just one big market or a single run. By splitting participants into separate, isolated groups (each with their own evolving download counts), they could directly observe that identical products and populations produced wildly different outcomes purely due to social feedback loops, not product quality or demographics.
Why it matters: Most experts (and many simulation practitioners) assume you just need one big, representative synthetic audience to get actionable results, but this study proves you need *many independent runs* to reveal true unpredictability and the risk of “false positives” (a hit that’s a fluke in one world, a flop in another). The method’s power comes from surfacing the *variance* across parallel histories, not just the average.
Example of use: If a company is using AI personas to test a new feature or ad campaign, they should run the experiment across several isolated groups (e.g., “virtual worlds” with no cross-talk or shared data) and compare the range of outcomes. This shows how much of a “hit” is luck versus a reliable effect—critical for launch decisions and risk assessment.
Example of misapplication: If the same company runs one giant simulation, or lets all personas see the same popularity signals, they might see a strong winner and assume it’s repeatable, missing the fact that in other parallel universes, a different product or message could have dominated. This could lead to overconfidence in a result that’s actually fragile and not robust to social feedback randomness.
🗺️ What are the Implications?
• Always account for social influence: Real-world consumer choices are heavily shaped by what others are doing, so studies that ignore peer effects or only measure isolated preferences will miss key drivers of market success.
• Run multiple parallel simulations: Even with identical products and starting conditions, outcomes can vary widely simply due to random early choices and social feedback. Running several “worlds” or simulations helps reveal the true range of possible results and reduces overconfidence in any single forecast.
• Stronger social signals increase both winners and unpredictability: Displaying popularity cues (like download counts or trending labels) makes bestsellers far more likely, but also makes it harder to predict which specific product will win. Consider how prominent social feedback is in your test design and adjust expectations accordingly.
• Product quality is not enough: While the worst products rarely become hits, many decent ones can fail and some mediocre ones can soar. Relying solely on expert judgment or quality ratings will not reliably pick winners—social dynamics must be included.
• Use inequality and unpredictability metrics: Track not just average outcomes but also the spread (e.g., Gini coefficient) and variation across runs to better understand and communicate market risks and volatility.
• Present results as ranges, not single predictions: Since outcomes are inherently unpredictable under social influence, share findings as confidence intervals or scenario ranges rather than exact rankings or scores.
• Factor in design elements that amplify social effects: Simple changes like showing popularity numbers or sorting by “most popular” can dramatically shift results—test with and without these features to understand possible market impacts.