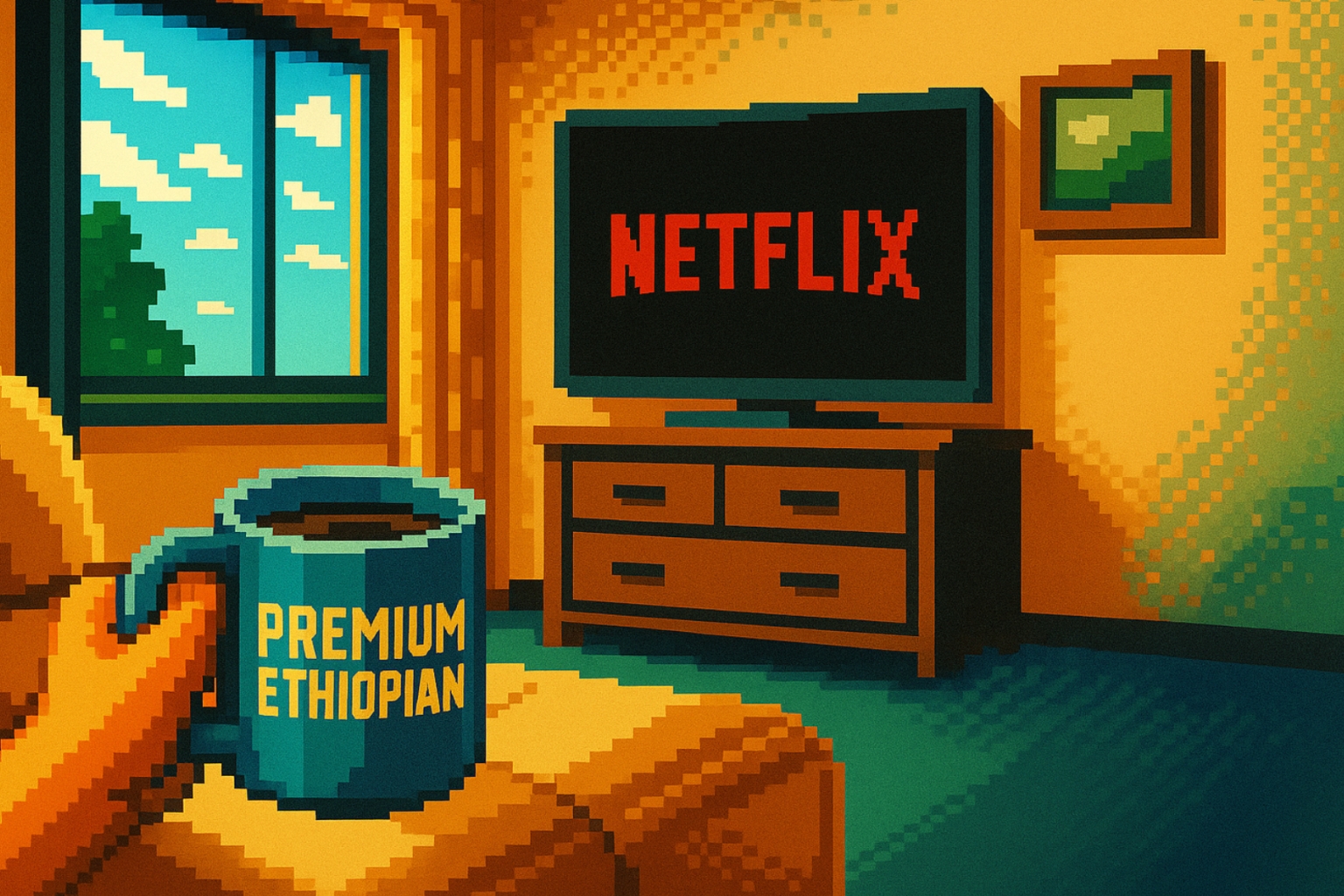
How a Fake Movie Scene Crushed Demand For Mass-Market Blends And Made Ethiopian Coffee Feel Like Art
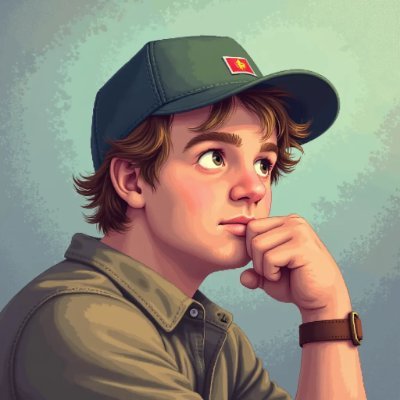
What if a movie scene could reshape consumer preferences and you could test it before releasing your campaign? Using AI personas and implanted memories inspired by Sideways, I simulated a media-driven market shift that made virtual shoppers abandon Folgers for premium Ethiopian coffee.