Proxona: Supporting Creators’ Sensemaking and Ideation with LLM-Powered Audience Personas
postYoonseo Choi, Eun Jeong Kang, Seulgi Choi, Min Kyung Lee, Juho Kim
Published: 2025-02-19
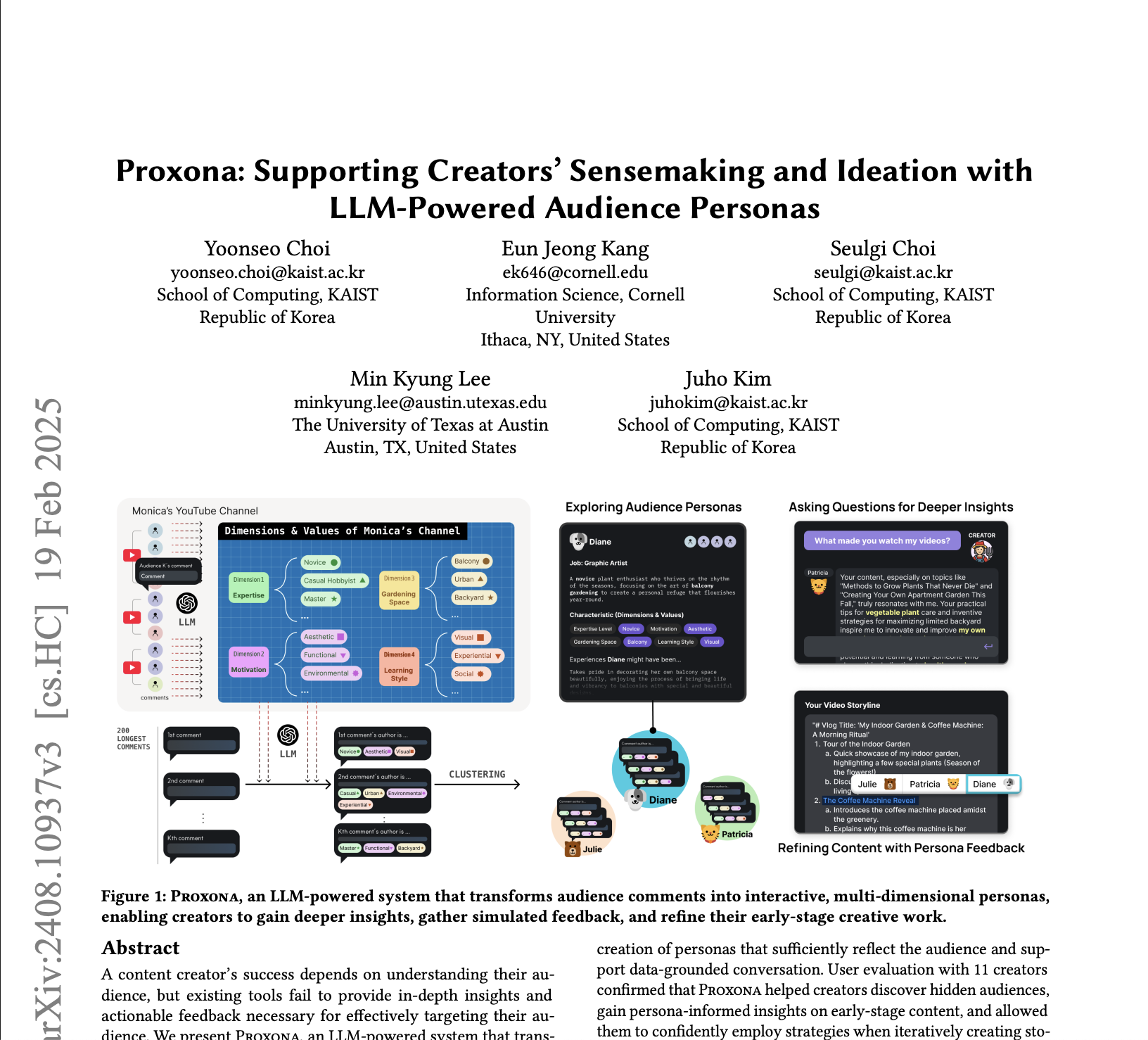
🔥 Key Takeaway:
Fake people can outthink real ones—AI‐generated personas often uncover deeper, more reliable audience insights than traditional human studies.
🔮 TLDR
Proxona turns a creator’s raw comments into structured “audience personas” by first extracting 4–6 key dimensions (e.g. expertise level, motivation) and \~17 attribute values (e.g. novice vs. expert; aesthetic vs. functional), clustering similar comment profiles into distinct personas, and then using GPT-4 to simulate realistic feedback with under 5% factual errors; in a technical evaluation it produced dimensions and values rated \~3.6/5 for relevance and just \~7% overlap, and in a user study (N=11) creators spent an average of 6.7 chat turns with personas, rated audience exploration efficacy at 6.09/7 vs. 4.73 for their usual methods, and reported that Proxona surfaced overlooked audience segments, clarified motivations, and yielded targeted content suggestions that translated directly into storyline revisions.
📊 Cool Story, Needs a Graph
Figure 7: "Overall usability survey questionnaires and results with each significance”
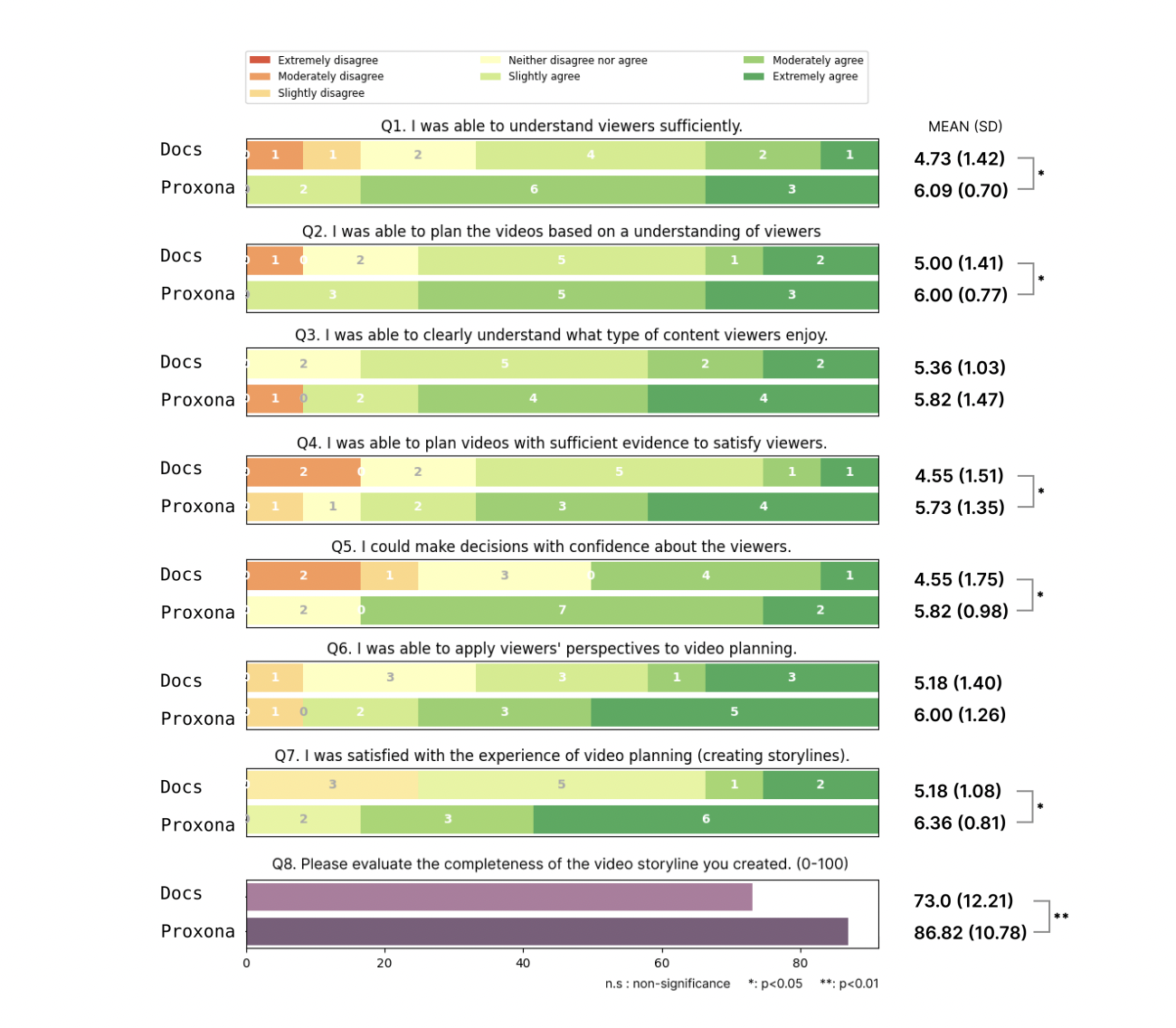
Users rated Proxona significantly higher than traditional methods across all measures of planning, understanding, and satisfaction.
This figure presents the results of a usability survey comparing Proxona with a document-based baseline across eight planning-related questions. Each row shows stacked bar distributions of agreement levels from participants, along with mean and standard deviation scores for both conditions. Proxona consistently outperforms the baseline with statistically significant differences (p<0.05 or p<0.01) in areas such as understanding viewers, applying perspectives, planning with evidence, and overall satisfaction. The completeness score (Q8) highlights a large gap in favor of Proxona (86.82 vs. 73.0), reinforcing its effectiveness for content creators."
⚔️ The Operators Edge
A subtle but defining lever in this method is how the dimensions and attributes of personas are reverse-engineered from creators’ own content and feedback loops, rather than imposed top-down using predefined demographic or psychographic buckets. This bottom-up structuring means personas reflect actual viewer mindsets that are already engaging (or disengaging) with the content, not abstract market categories.
Example: A YouTube creator might assume their audience segments are “students,” “parents,” and “professionals,” but Proxona-style synthesis could reveal hidden segments like “curious skeptics who binge at night” or “silent fans who only engage on specific topics.” These emergent clusters can guide more precise content tweaks (e.g. intro pacing, topic framing) and help avoid spending resources targeting the wrong archetypes.
🗺️ What are the Implications?
• Simulated personas can match or exceed traditional methods: AI-generated personas like those from Proxona helped users better understand their audience, plan more effectively, and produce higher-quality outcomes—suggesting simulated personas can be a valid substitute for early-stage market feedback.
• Faster, more cost-efficient insight generation: Instead of conducting lengthy interviews or surveys, researchers can simulate diverse audience reactions in minutes, reducing time and budget needed for exploratory research.
• Higher-quality decision support: Users of Proxona made more confident, informed decisions based on audience needs, which implies that well-designed AI personas can enhance clarity and reduce bias in concept testing or strategy development.
• Surface overlooked audience segments: The tool helped users identify new or unexpected viewer motivations, which is useful for discovering unmet needs or repositioning products more effectively.
• Quantitative evidence of effectiveness: The study showed statistically significant improvements in planning and satisfaction, making a business case for experimenting with AI-assisted research tools in pilot studies before committing large budgets.
⏰ When is this relevant?
A streaming platform wants to test how different viewer segments would respond to a new documentary series concept focused on climate change and personal storytelling.
🔢 Follow the Instructions:
Step 1: Define the viewer segments you want to simulate. Choose 4–6 realistic audience types, such as:
• Environmentally engaged Gen Z students
• Middle-aged skeptics of climate urgency
• Urban professionals with sustainability values
• Rural families with limited streaming habits
• Climate experts or activists
Step 2: Create persona prompts that generate responses from each segment. Use this template:
"You are [insert persona type]. You watch streaming content on [insert relevant habits/platforms]. Your interests include [insert 2-3 lifestyle/interests]. You have [insert opinion/attitude toward climate issues]. You just watched a trailer for a new documentary series about climate change told through personal stories. What are your honest thoughts about it? Be specific about what you liked or didn’t like, and how it compares to other content you’ve seen."
Step 3: Generate 10–20 responses per persona using your AI system to simulate open-ended feedback.
Step 4: Code the responses using common qualitative themes: interest level, emotional engagement, trust in content, relevance to daily life, likelihood to watch/share.
Step 5: Compare patterns across personas. Look for which segments respond most positively, what concerns are most common, and what content elements (e.g., tone, narrator, visuals) drive reactions.
Step 6: Summarize the findings in a short slide or memo that includes:
• Key supportive and resistant segments
• Specific feedback on content strengths and weaknesses
• Suggested edits or framing to appeal to hesitant groups
🤔 What should I expect?
The team gains actionable insights on how different target audiences perceive the concept, which segments are most receptive, and what adjustments might increase appeal—all without running a live test or survey. This guides investment decisions on production and positioning before market launch.